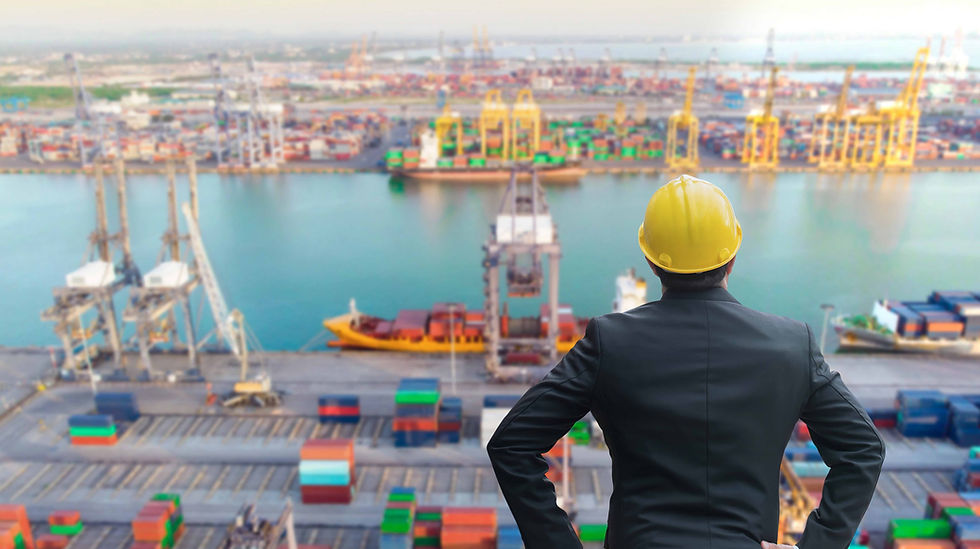
Reality and real-world data never cease to amaze me. A recent customer experience underscored just how surprising and insightful real-world data can be.
The First Signs of Trouble
It started with an urgent call from Mark, the operations manager at one of our largest port terminal customers.
"Something's wrong with your system," Mark said firmly.
"The failure rate comparison between our Terminal Tractors doesn't make any sense. These are identical machines with nearly identical usage patterns."
I pulled up his dashboard while we spoke. "Can you walk me through what you're seeing that concerns you?"
"Look at Tractors 5 and 8. They're the same model, same year, same everything. But your system shows Tractor 8 failing twice as often. That can't be right."
Digging Into the Mystery
I immediately connected Mark with our support team lead, Adina, who spent several hours reviewing the data with him. Ten seemingly identical Terminal Tractors, each approximately six years old.
"I've looked at three years of operational data covering 7,662 shifts," Adina explained during their follow-up call.
"These tractors experienced a total of 1,850 failures, but the distribution is indeed unusual. The failure rates range dramatically from 0.3 to over 0.6 failures per shift."
"This data can't be right," Mark insisted, frustration evident in his voice. "We purchased these tractors in the same batch. They should be performing similarly."
Adina assured him, "I understand your concern. Let me escalate this to our data science team. We'll figure out if there's a bug in our analysis."
The Investigation Deepens
Our data science team spent a week diving deep into not just Mark's data, but similar patterns across our customer base. I joined the call when they were ready to present their findings to Mark.
"We've triple-checked everything," explained Boaz, our lead data scientist.
"We compared your data with similar equipment from five other terminals.
The variance you're seeing isn't a bug, it's actually a real phenomenon."
"That's impossible," Mark replied. "How could identical machines vary so dramatically?"
Boaz shared his screen, showing several detailed charts. "Look at these patterns. The tractors with higher failure rates show distinct operational signatures. It's not about the tractors themselves, it's about how they're being used."
The Surprising Reality
"Wait, are you saying some of our operators are breaking the tractors?" Mark asked, his tone shifting from frustration to curiosity.
"Not intentionally," I clarified. "But we've identified three key factors that are creating these differences."
We observed consistent patterns and behaviors across multiple customers and equipment types, highlighting real-world factors like:
Operator Training and Behavior: "The data shows that Tractors 5 and 8 are primarily operated by your newer staff," Boaz explained. "We can see subtle differences in acceleration patterns and braking behavior that create additional strain."
Workload Intensity: "Terminal 3 East, where you keep Tractors 5 through 8, handles 40% more container movements per shift," I added. "That higher workload leads directly to accelerated equipment wear, especially on the transmission systems."
Manufacturing Quality: "Even minor differences in materials or assembly can have outsized effects on reliability," Boaz continued. "Batch variations that seem insignificant on paper can compound over thousands of operational hours."
The Transformation
Mark went silent for a moment before responding: "So you're telling me that how we use the tractors matters just as much as, or even more than, how old they are?"
"Exactly," I confirmed. "Age is just one factor among many. Your operational patterns and driver behaviors are creating these differences."
"This... this changes everything about how we manage our fleet," Mark said, his voice reflecting the weight of this realization.
Nine weeks later, Mark called back with excitement in his voice. "We implemented those changes you suggested, rotating operators between tractors, smart equipment assignment and dispatching based on its risk of breakdown during operations, and adjusting our maintenance schedules based on usage patterns rather than just time intervals."
"And?" I asked, already suspecting the answer.
"Failures are down approximately 18% across the board," he said triumphantly. "I've never seen anything like it. My boss thinks I'm some kind of miracle worker now."
The Bigger Lesson
When we first built our platform, we assumed equipment of the same type and age would perform similarly.
Mark's "bug report" revealed something far more valuable, a counterintuitive truth that how equipment is used is just as important as the equipment itself.
Using this insight, Mark's team implemented targeted preventive maintenance, refreshed operator training, and enhanced monitoring to address these challenges proactively.
What started as a customer complaint ended as one of our most valuable discoveries. Sometimes the most important insights come disguised as errors, reminding us to stay curious about the stories data tells us, especially when they challenge our assumptions.